Deepfake A Big Issue : Evolution of Deepfake Technology
Deepfake technology refers to the use of artificial intelligence, specifically deep learning, to create or manipulate video and audio content, making it possible to fabricate realistic-looking footage of people saying or doing things that they never actually did. The term “deepfake” comes from the combination of “deep learning” and “fake.” This technology leverages neural networks, which mimic the human brain’s ability to recognize patterns and learn from data.
The evolution of deepfake technology began in the early 2010s, with significant advancements occurring around 2017. The technology initially required substantial computational power and expertise, limiting its use to those with access to high-end resources. However, by 2018, user-friendly deepfake applications became accessible, lowering the barrier to entry and enabling widespread use.
Deep Learning Techniques Used in Deepfake Creation
Deepfake creation primarily involves two deep learning techniques: autoencoders and generative adversarial networks (GANs). Autoencoders are used to compress and decompress images, helping in swapping faces in videos. GANs involve two neural networks, a generator and a discriminator, which work against each other to create increasingly realistic images or videos.
- Autoencoders: Used for encoding (compressing) and decoding (decompressing) the images, especially in face-swapping applications.
- Generative Adversarial Networks (GANs): Utilized for generating new, synthetic instances of data that can pass for real data, enhancing the realism of deepfakes.
The Rising Prevalence and Accessibility of Deepfake Tools
Deepfake technology has become increasingly prevalent and accessible. By 2019, numerous deepfake tools were available for public use, making it possible for individuals without extensive technical knowledge to create deepfakes. The trend continued into 2020 and 2021, with more sophisticated and user-friendly tools emerging, including mobile applications. This ease of access has raised concerns about the potential misuse of deepfakes in misinformation, entertainment, and other domains.
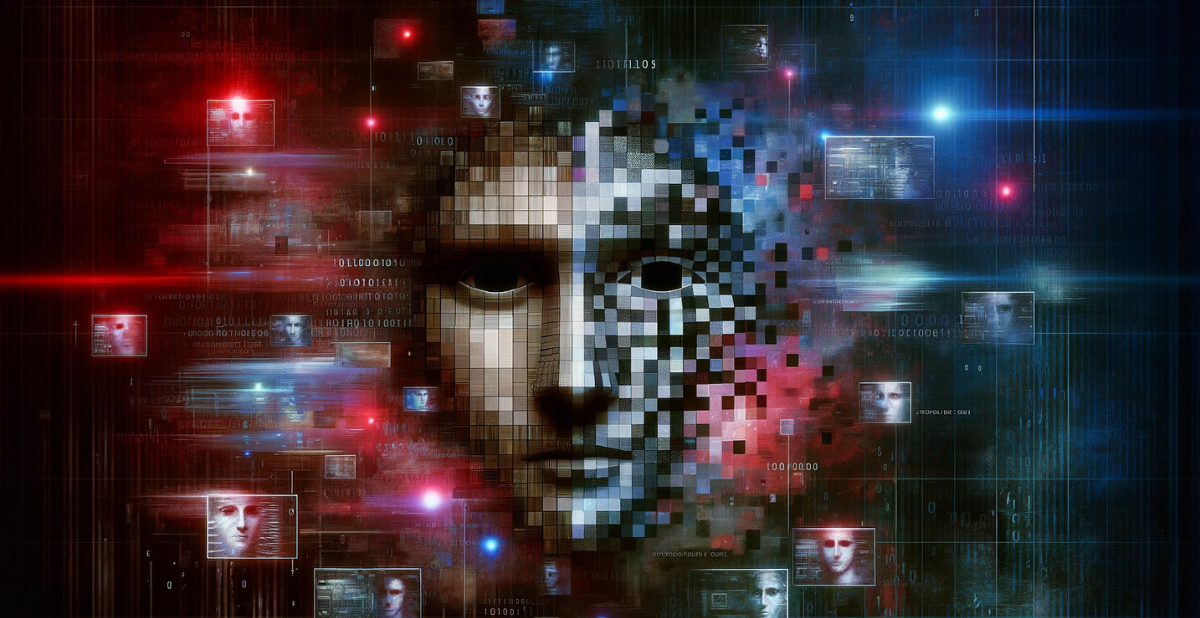
The Impact of Deepfakes on Social Media Platforms
Deepfake technology has significantly impacted social media platforms, prompting various responses and measures to detect and regulate deepfake content. These platforms face challenges in balancing the freedom of expression with the prevention of misinformation and harmful content.
Twitter’s Response and Measures Against Deepfakes
In February 2020, Twitter announced a policy specifically targeting manipulated media, including deepfakes. Key aspects of this policy include:
- Labeling Tweets: Tweets containing manipulated media are labeled to provide context.
- Warning Messages: Before a user retweets, likes, or replies to such content, a warning message is displayed.
- Reduced Visibility: Twitter may reduce the visibility of these tweets.
- Removal of Content: Content that is likely to cause harm, such as threats to physical safety or election integrity, is subject to removal.
Facebook’s Initiatives and Deepfake Detection Challenges
Facebook has taken several initiatives to combat deepfakes:
- Partnership with Academia and Industry: In September 2019, Facebook launched the Deepfake Detection Challenge in collaboration with academic institutions and other organizations to improve the detection of deepfakes.
- Banning Deepfakes: In January 2020, Facebook announced a policy to remove deepfakes that are misleading and not parody or satire.
- Investment in Detection Technologies: Facebook has invested in developing better detection technologies to identify deepfakes.
Despite these efforts, Facebook faces challenges in accurately detecting deepfakes due to the evolving nature of the technology and the need to respect user privacy and freedom of expression.
The Stance of Other Social Media Platforms
Other platforms have also addressed deepfake content:
- Reddit: Banned deepfakes in February 2018, particularly those that are non-consensual or misleading.
- Gfycat: Has a policy against deceptive deepfakes and actively removes such content.
- Pornhub: Banned deepfake pornographic content in February 2018, citing its non-consensual nature.
These platforms recognize the potential harm caused by deepfakes, especially in spreading misinformation and violating personal consent, and have implemented policies to mitigate these issues.
Deepfake Detection Techniques
Deepfake detection is a rapidly evolving field, encompassing a variety of methods and technologies to identify audio and video manipulations. These techniques range from conventional digital forensics to innovative machine learning algorithms, and the emerging use of blockchain and digital signing for verification.
Audio and Video Deepfake Detection Methods
- Visual and Audio Analysis: This involves scrutinizing videos and audio recordings for inconsistencies or anomalies. Visual analysis looks for irregularities in facial expressions, lip-syncing errors, and unnatural eye movements. Audio analysis focuses on detecting voice inconsistencies and unnatural speech patterns.
- Machine Learning Models: Advanced machine learning models, particularly those using deep learning techniques, are trained to differentiate between genuine and fake content. These models analyze various features of media files, including texture, noise patterns, and biological signals like heart rate (visible through subtle skin color changes).
- Forensic Methods: Digital forensic techniques involve examining the metadata of the media files for clues about their origin or alterations. This includes scrutinizing file formats, compression artefacts, and editing traces.
Innovative Approaches and Algorithms for Deepfake Identification
- Convolutional Neural Networks (CNNs): CNNs are effective in analyzing visual data and identifying subtle patterns indicative of deepfakes.
- Recurrent Neural Networks (RNNs): RNNs, particularly those using Long Short-Term Memory (LSTM) units, are used for audio deepfake detection, analyzing speech patterns over time.
- GAN Fingerprinting: As many deepfakes are created using GANs, some detection methods involve identifying unique ‘fingerprints’ left by specific GAN architectures.
- Biometric Analysis: This includes the use of heart rate detection and micro-expression analysis to identify inconsistencies in video content.
The Role and Effectiveness of Blockchain and Digital Signing in Deepfake Verification
- Blockchain Technology: Blockchain offers a decentralized and tamper-proof ledger, which can be used to authenticate the origin and integrity of digital content. By storing the digital signatures or hashes of original content on a blockchain, it becomes possible to verify if a piece of media has been altered.
- Digital Signing: Digital signing involves attaching a cryptographic signature to media content at the time of creation. This signature can later be used to verify the content’s authenticity and integrity.
- Effectiveness: While blockchain and digital signing are promising for verification, they require widespread adoption and standardization. Their effectiveness is contingent on the original content being signed and recorded, which is not always feasible or practical.
In summary, deepfake detection is a multifaceted challenge that incorporates various techniques from digital forensics to advanced machine learning. The effectiveness of these methods varies, and no single approach is foolproof. The incorporation of blockchain and digital signing presents a forward-looking solution, but its practical implementation remains a challenge due to the need for broader adoption and standardization.
Legal and Ethical Considerations
The rise of deepfake technology has spurred legal and ethical debates, leading to various actions and legislation across countries, and highlighting the intricate balance between advancing technology and protecting privacy.
Legal Actions and Legislation Against Deepfakes in Various Countries
Country | Legislation/Action | Description |
---|---|---|
USA | DEEPFAKES Accountability Act (Introduced in 2019) | Proposes to criminalize the malicious creation and distribution of deepfakes. Includes requirements for labeling deepfakes and consent from individuals depicted. |
UK | Various existing laws (e.g., defamation, harassment) | While there’s no specific law for deepfakes, existing laws cover aspects like harassment, defamation, and privacy breaches. |
China | New regulations (Effective from 2020) | Mandates that all content created using AI or VR must be clearly marked, with violations potentially leading to criminal charges. |
Ethical Dilemmas and the Balance Between Technology and Privacy
- Consent and Privacy: The creation of deepfakes often occurs without the consent of the individuals depicted, raising significant privacy concerns.
- Freedom of Expression vs. Harm Prevention: Balancing the right to freedom of expression with the need to prevent harm caused by deceptive deepfakes is a key ethical challenge.
- Misinformation and Trust: Deepfakes can be used to spread misinformation, undermining public trust in media and institutions.
Challenges in Legislating Against Deepfakes and the Role of International Cooperation
- Technological Evolution: The rapid advancement of deepfake technology makes it challenging for legislation to keep pace.
- Jurisdictional Issues: Deepfakes can be created and distributed across borders, making it difficult for national laws to be effective in isolation.
- Free Speech Concerns: Legislating against deepfakes must balance the prevention of harm with the protection of free speech.
- International Cooperation: Addressing the global nature of deepfakes requires international cooperation in creating and enforcing laws, and in sharing best practices and technological solutions.
the legal and ethical landscape surrounding deepfakes is complex and evolving. Countries are enacting legislation and taking legal actions to combat the malicious use of deepfakes, but face challenges in keeping pace with technological advances, ensuring jurisdictional effectiveness, and balancing ethical dilemmas. International cooperation plays a crucial role in creating a unified approach to this global issue.
Deepfake in Popular Culture and Media
Deepfake technology has permeated popular culture and media, being utilized in films, TV shows, and music videos. Its portrayal has both increased awareness and raised questions about the ethical implications of such technology.
Historical and Recent Examples of Deepfake in Films, TV Shows, and Music Videos
Year | Title | Medium | Description |
---|---|---|---|
1994 | Forrest Gump | Film | Early example of digital manipulation, inserting the protagonist into historical footage. |
2016 | Rogue One: A Star Wars Story | Film | Used CGI to recreate late actor Peter Cushing and a younger Carrie Fisher. |
2019 | Gemini Man | Film | Featured a digitally de-aged Will Smith, showcasing advanced visual effects. |
2020 | The Mandalorian | TV Show | Used deepfake-like technology to bring a young Luke Skywalker to life. |
2021 | WandaVision | TV Show | Employed deepfake techniques for character transformations and effects. |
Music Videos | Music Industry | Artists like Billie Eilish and Taylor Swift have used deepfake technology for creative effects in their music videos. |
The Portrayal and Awareness of Deepfake Technology in Popular Culture
- Creative Uses: In film and TV, deepfake technology has been used to de-age actors, resurrect historical figures, and create complex visual effects, showcasing its creative potential.
- Ethical Questions: The use of deepfakes in media raises questions about consent, especially when recreating images of deceased individuals or altering living actors’ appearances.
- Awareness and Debate: Popular culture’s adoption of deepfakes has sparked public discussion about the technology’s capabilities and potential misuse, increasing general awareness.
- Dystopian Themes: Some films and TV shows have portrayed deepfakes within dystopian narratives, highlighting concerns about misinformation and identity theft.
Challenges and Solutions to Deepfake Technology
The challenges posed by deepfake technology are multifaceted, involving technical, ethical, and legal aspects. Addressing these challenges requires the concerted efforts of various stakeholders, each playing a crucial role in developing effective solutions.
The Complexity of Mitigating Deepfake Impacts
- Technological Advancements: Keeping pace with the rapid advancement of deepfake technology.
- Detection Difficulties: Developing reliable methods to detect deepfakes, as the technology becomes increasingly sophisticated.
- Ethical and Legal Issues: Balancing the ethical use of deepfakes with freedom of expression and privacy concerns.
Roles of Various Stakeholders in Addressing Deepfake Challenges
Stakeholder | Role | Description |
---|---|---|
Technical Experts | Development and Research | Innovating advanced detection methods and improving public understanding of deepfakes. |
Social Media Platforms | Policy Implementation | Implementing policies to identify and regulate deepfake content on their platforms. |
Policymakers | Legislation and Regulation | Creating laws and regulations to prevent malicious use of deepfakes and protect individual rights. |
Educational Institutions | Awareness and Training | Educating the public and future professionals about the implications of deepfake technology. |
Media Organizations | Reporting and Fact-Checking | Reporting responsibly on deepfake incidents and investing in fact-checking resources. |
Prospects for Future Technology and Policy Development
- Advanced Detection Technologies: Continued development of more sophisticated detection tools, potentially leveraging AI and machine learning.
- International Collaboration: Global cooperation in standardizing policies and sharing best practices for deepfake regulation.
- Public Education and Awareness: Increasing public awareness about the nature of deepfakes and how to critically evaluate digital content.
- Ethical Guidelines: Establishing industry-wide ethical guidelines for the responsible use of deepfake technology.
addressing the challenges posed by deepfake technology requires a multifaceted approach, involving technical innovations, policy development, public education, and international cooperation. The roles of various stakeholders are critical in formulating and implementing effective solutions to mitigate the impacts of deepfakes while considering the ethical and legal implications. The future prospects in this field are geared towards advanced detection methods, stronger international policies, and heightened public awareness.